About Us
The UCLA Astrophysics Data Lab aims to:
- Answer fundamental questions in astrophysics using the increasingly large datasets that are available now and in the future.
- Build the framework for translating machine learning methods to astrophysics.
- Develop innovative ways of using data for astrophysics.
- Help astronomers integrate new data-driven methods and practices into their work.
Cosmology with machine learning
Scientifically, the Lab is currently concentrating on probabilistic neural networks as a method for improving photometric redshifts to measure dark energy with the upcoming LSST survey with the Vera Rubin Observatory. The nature of dark energy is one of the biggest mysteries in physics and astrophysics as it represents 72% of the energy density of the Universe but we have very few constraints on its physical origin.
Because dark energy affects the fabric of the Universe, the LSST survey will image over 5 billion galaxies allowing us to see the effects of dark energy on the distribution of matter at unprecedented precision. For this project to be successful, we need to know the distances to all these galaxies and understand the uncertainty of our measurements.
Because galaxies are very complex objects, the most promising methods involve using machine learning to empirically learn about how galaxies vary across cosmic time. The biggest challenge for these machine learning models is to have accurate uncertainty estimates. Our novel approach to this problem is to use probabilistic neural networks, which are like more typical neural networks but can propagate uncertainties throughout the model.
Probabilistic deep learning such as Bayesian Neural Networks has many advantages compared to traditional neural networks, including: better uncertainty representations, better point predictions, and offers better interpretability of neural networks because they can be viewed through the lens of probability theory. In this way we can draw upon decades of development in Bayesian inference development. We think that probabilistic neural networks have the potential to be a transformative tool for cosmology by embedding both physics of the universe and telescope and instrumental characteristics into the same neural network.
The human side of machine learning
An important aspect of the Astrophysics Data Lab is to adapt machine learning tools from industry for scientific purposes and to help develop best practices in their use. We are currently investigating the following research questions: (1) how do new computational capabilities change practices of doing science? (2) What knowledge and skills are needed to shift to new computational environments? (3) How do training, labor, credit, buy-in and rewards accelerate or hinder transitions to new workflows?
To answer these questions, have been interviewing astronomers using machine learning to understand their motivation for using machine learning and how they are using it in their own work. We have interviewed astronomers from around the world and at different stages in their career including grad students, postdocs, and faculty.
We have also done several projects aiming at replicating machine learning papers to understand the issues involved in machine learning workflows and how to preserve machine learning models. We are currently preparing a paper on this work and aim to disseminate it at machine learning conferences focusing on the physical sciences.
Recent Products and Publications
Products
GalaxiesML - a public dataset of galaxy images, photometry, redshifts, and structural parameters for machine learning.
Recent Publications
- Learning the Evolution of Physical Structure of Galaxies via Diffusion Models, Lizarraga A., Hanchen Jiang E., Nowack J., Li Y. Q., Nian Wu Y., Boscoe B., Do T., 2024,
Neurips Machine Learning and the Physical Sciences, 2024, arXiv:2411.18440
- Using different sources of ground truths and transfer learning to improve the generalization of photometric redshift estimation, Soriano J., Saikrishnan S., Seenivasan V., Boscoe B., Singal J., Do T., 2024,
Neurips Machine Learning and the Physical Sciences, 2024, arXiv:2411.18054
- Redshift Prediction with Images for Cosmology Using a Bayesian Convolutional Neural Network with Conformal Predictions, Jones E., Do T., Li Y. Q., Alfaro K., Singal J., Boscoe B., 2024,
The Astrophysical Journal, 974, 159
- GalaxiesML: a dataset of galaxy images, photometry, redshifts, and structural parameters for machine learning, Do T., Boscoe B., Jones E., Li Y. Q., Alfaro K., 2024,
arXiv:2410.00271
- Using Galaxy Evolution as Source of Physics-Based Ground Truth for Generative Models, Li Y. Q., Do T., Jones E., Boscoe B., Alfaro K., Nguyen Z., 2024,
arXiv:2407.07229
- Improving Photometric Redshift Estimation for Cosmology with LSST Using Bayesian Neural Networks, Jones E., Do T., Boscoe B., Singal J., Wan Y., Nguyen Z., 2024,
The Astrophysical Journal, 964, 130
- Elements of effective machine learning datasets in astronomy, Boscoe B., Do T., Jones E., Li Y., Alfaro K., Ma C., 2022,
arXiv:2211.14401
- Machine Learning Classification to Identify Catastrophic Outlier Photometric Redshift Estimates, Singal J., Silverman G., Jones E., Do T., Boscoe B., Wan Y., 2022,
The Astrophysical Journal, 928, 6
- Photometric Redshifts for Cosmology: Improving Accuracy and Uncertainty Estimates Using Bayesian Neural Networks, Jones E., Do T., Boscoe B., Wan Y., Nguyen Z., Singal J., 2022,
arXiv:2202.07121
- Elements of effective machine learning datasets in astronomy, Bernie Boscoe, Tuan Do, Evan Jones, Yunqi Li, Kevin Alfaro, Christy Ma, 2022,
Neurips Machine Learning and the Physical Sciences 2022, arXiv:2202.07121
List of our group papers: ADS Link
Members
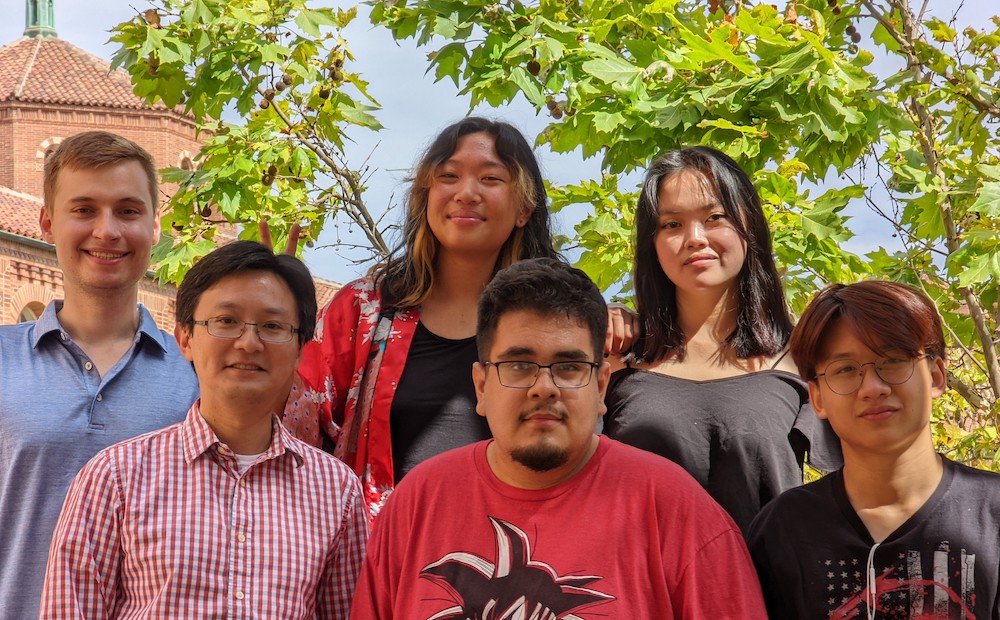
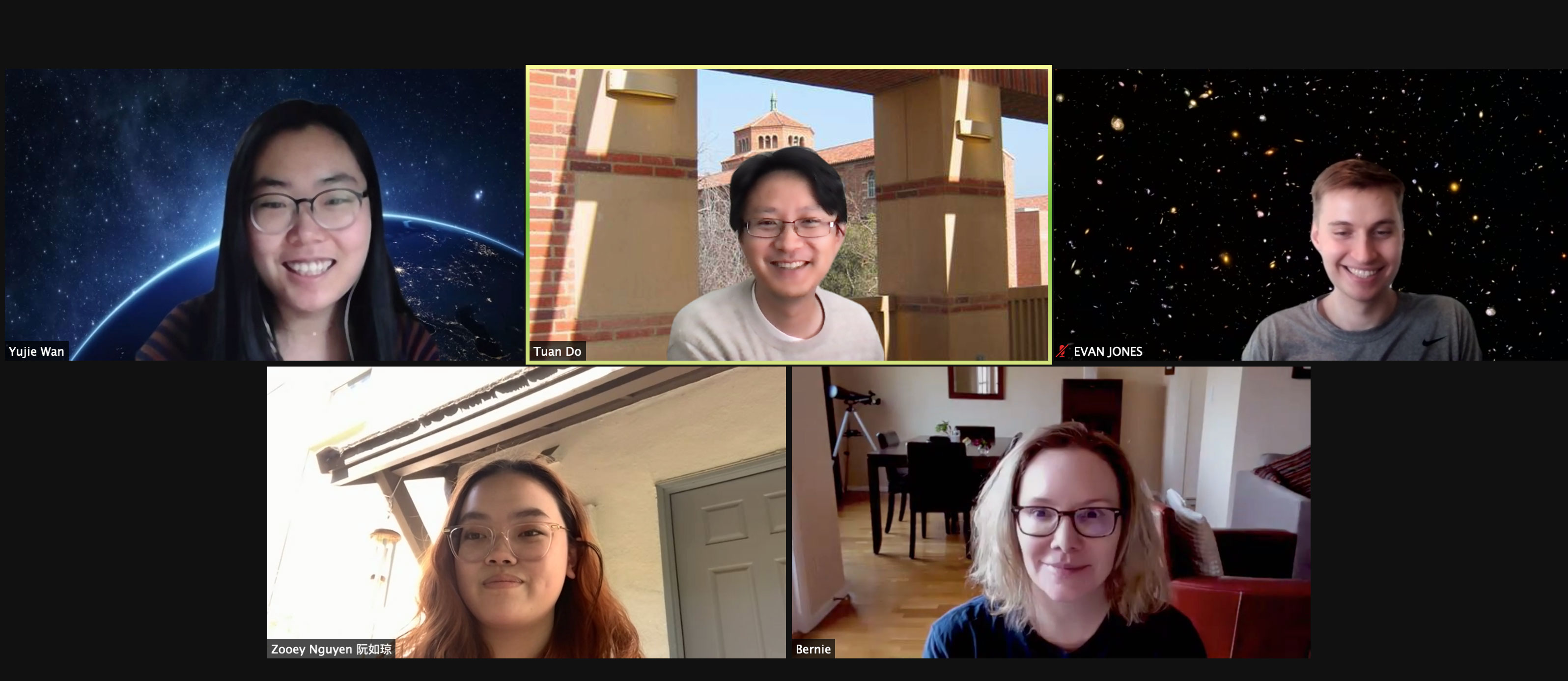
- PI: Prof. Tuan Do (UCLA)
Associate Professor, Physics and Astronomy
Office: PAB 3-945, Phone: 310-794-9466
Email: tdo@astro.ucla.edu
Website - Prof. Bernadette (Bernie) Boscoe
Assistant Professor at Southern Oregon University (SOU)
Email:boscoeb@sou.edu
Website - Jonathan Soriano
UCLA graduate student - Chandler Campbell
Southern Oregon University Research Software Engineer - Jacob Nowack
SOU undergraduate student - Srinath Saikrishnan
UCLA undergraduate student - Vikram Seenivasan
UCLA undergraduate student
- Bernie Boscoe (postdoc)
- Evan Jones (grad student)
- Hang Min
- William Chang, currently at Yale
- Yun Qi (Billy) Li, currently at University of Washington
- Christy (Chris) Ma, currently at University of Pennsylvania
- Kevin Alfaro
- Nissia Indradjaja
- Yujie Wan, currently at University of Illinois, Urbana-Champaign
- Zooey Nguyen
UCLA Graduate students - please contact Prof. Do for details.
UCLA Undergraduates - our lab takes on new research students through the Physics and Astronomy Department Summer Undergraduate Research Program or the UC Leads program. Applications for these programs are due in Winter or Spring each year.
Students not from UCLA - unfortuntately, we do not have the capacity to mentor students not from UCLA.